Artificial intelligence is no longer a futuristic concept, it's a tangible driver of business transformation today. Yet, many companies still struggle with how to approach AI implementation effectively.
In a recent episode of the TEQ Shift Podcast, Boris Bialek shared his hands-on experience with AI projects across industries. From helping banks and retailers to optimising medical research workflows, he emphasises a structured, value-driven approach to leveraging AI.
Here’s a breakdown of the key insights and real-world use cases discussed, guiding you on how to build practical AI solutions that deliver real business value.
1. Start with the Business Problem, Not the Tech
The biggest mistake companies make when starting with AI is focusing on technology first. Leaders often get caught up in tools, forgetting the fundamental question: "What problem are we solving?"
“Start with one thing: the business, not the technology.”
To get it right, Boris recommends starting with a clear business objective. Define what you are trying to achieve—whether it’s faster data processing, cost reduction, or enhanced customer interaction. Only then should you map out the technical path.
Example: Novo Nordisk’s Clinical Reports
Novo Nordisk, a leading pharmaceutical company, faced exactly this challenge with clinical study reports. These critical documents took medical professionals up to 15 weeks to complete. Despite years spent trying to streamline this, the process only got slower. By reframing the issue as a business challenge first, Novo Nordisk collaborated with MongoDB to implement an AI solution using LangChain’s NLP, AWS Bedrock, and MongoDB’s vector search. The result? Report generation time dropped dramatically—from 15 weeks to just 10 minutes.
Action step: Map out your problem first. Use workshops with business stakeholders to align on desired outcomes before selecting your AI tools.
2. First, Clean Up Your Data
Jumping into AI without a data strategy is a recipe for failure. Data is often siloed, messy, or tied up in legacy systems. AI models are only as good as the data you feed them.
“If you don’t clean up your data, don’t start with AI.” — Boris Bialek
Start by identifying where your data lives and what shape it’s in. Are you dealing with structured, semi-structured, or unstructured data? Consider both data quality and accessibility before starting your AI project.
Example: Lombard Odier’s Legacy Systems
This financial institution faced performance bottlenecks due to legacy data structures. By modernising their data architecture, they made real-time analytics and AI integration feasible.
Action step: Audit your data before you build. Clean, integrate, and structure it to enable efficient AI application.
3. Prioritise Real-Time Capabilities
One of the most common pitfalls in AI projects is underestimating the need for real-time processing. Chatbots, predictive maintenance, and personalised recommendations are just a few areas where speed is essential.
Example: Real-Time Chatbots in Banking
Boris pointed out that some companies build chatbots with a 15-second response time—far too slow for modern expectations. Integrating vector searches and real-time data pipelines helped reduce response times to milliseconds.
Action step: Assess your speed requirements upfront. Consider whether your use case demands instant responses or can tolerate latency.
4. Choose the Right Infrastructure
AI projects require flexible and scalable infrastructures. Boris emphasises the importance of matching the technology to the use case rather than forcing a one-size-fits-all solution.
Example: Retail Logistics with MongoDB
When working with a retailer to optimise stock management, Boris and his team built a model that processed 10 million items per hour, saving over 600 million in logistics costs annually. The key was using MongoDB’s JSON model for flexible data handling.
Action step: Don’t lock yourself into one tool. Use a modular architecture that can evolve as your needs change.
5. Integrate AI with Legacy Systems
Modernisation is a gradual process. AI initiatives often fail because they don’t account for existing systems and how to integrate with them.
Example: Document Handling in Financial Services
Boris discussed how AI can turn archival data into active datasets. In financial institutions, this means extracting valuable insights from old client records and compliance files, rather than just archiving them.
Action step: Build integration points between new AI modules and existing data ecosystems to ensure continuity.
Final Thoughts: Think Big, But Start Smart
The AI landscape is rapidly evolving. While it’s tempting to aim for disruptive innovation, practical, incremental improvements often yield more reliable business value. As Boris summarises:
“Think big, but don’t be afraid to start with small, manageable steps.”
By combining clear problem statements, clean data, real-time capabilities, and robust QA, you can unlock AI’s potential without falling into common traps.
Want to dive deeper into real-world AI transformation? Listen to the full episode with Boris Bialek on the TEQ Shift Podcast.
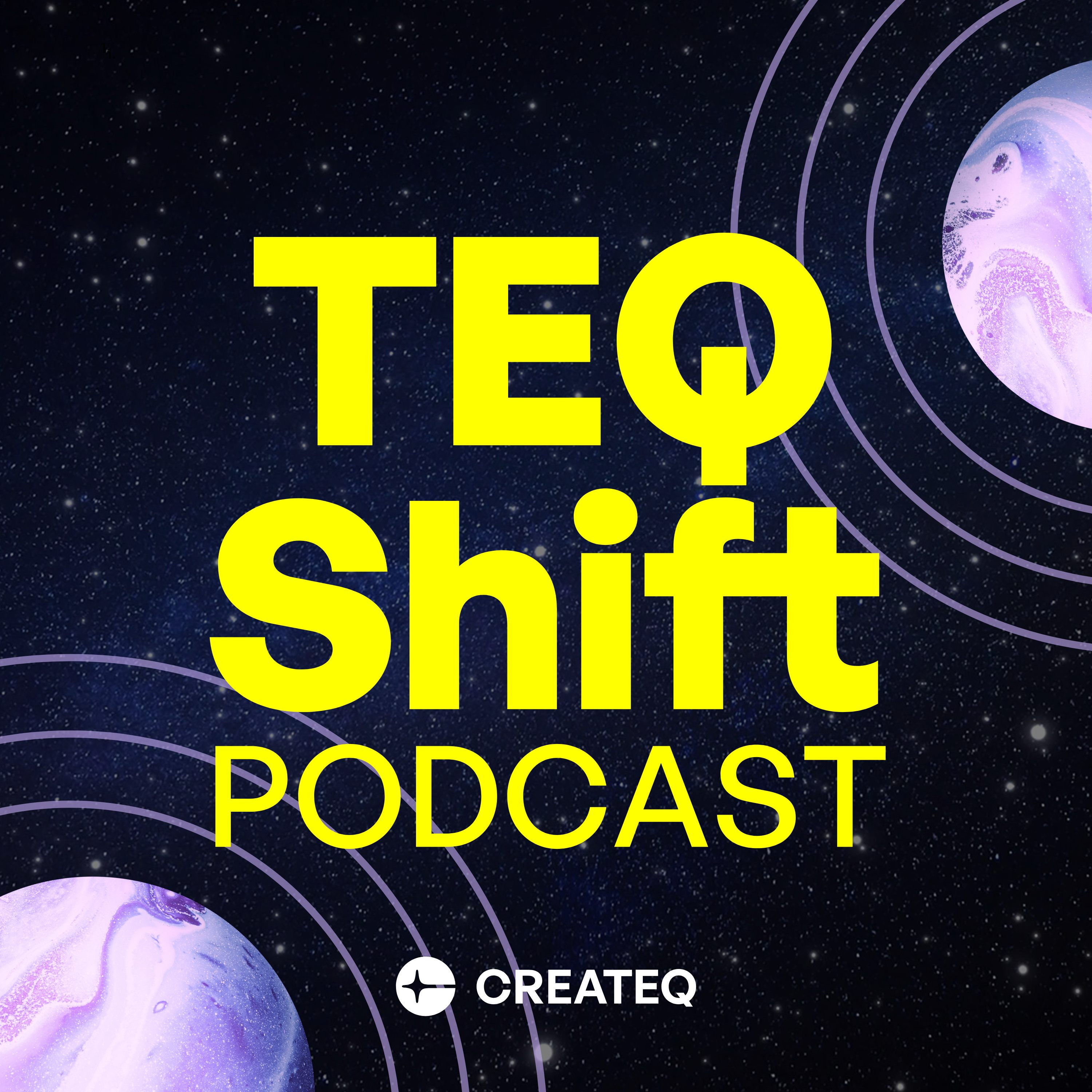